SARA E. HANSEN

Machine Learning
Species distribution models (SDMs) are a powerful tool for addressing urgent ecological issues like the spread of invasive species. Continually refining the process of building and applying SDMs can ensure the decisions resulting from them are well supported and biologically informed. Using the invasive aquatic plant European frog-bit as a case study, I explored five decision points across the SDM pipeline to explore how choices made by modelers in data processing and data selection influence model end results and possible interpretations. I built SDMs using twelve machine learning, regression, and envelope modeling algorithms and analyzed relative model performance, contributions of each explanatory variables, and prediction values. The study is fully reproducible and open-access.
​
Get the code from Open Science Framework or GitHub
​
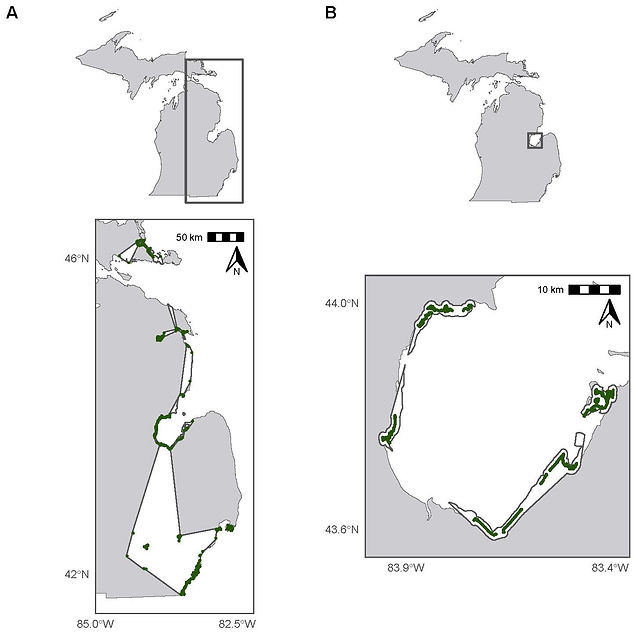
